Research Fellow in Deep Learning in Medical Image Computing and Modelling
The University of Leeds, School of Computing, has up to two vacancies for a Research Fellow in Deep Learning in Medical Image Computing and Modelling
- Closing date: 30 Nov 2020
- United Kingdom | University of Leeds
- Date posted: 19 Nov 2020
- Job type: Academic: postdoc
- Discipline: Computational science & software engineering
Employer profiles
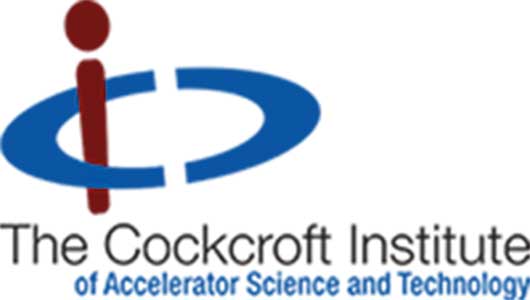
The Cockcroft Institute of Accelerator Science and Technology
The Cockcroft Institute is a partnership between the universities of Lancaster, Liverpool, Manchester and Strathclyde and the Science and Technology Facilities Council, devoted to the development and construction of particle accelerators and intense sources of radiation for pure and applied research. The Institute – a collaboration between academia, national laboratories, industry and the local economy – brings together the best accelerator scientists, engineers, educators and industrialists to conceive, design, construct and use innovative accelerator-based instruments of discovery at all scales and lead the UK’s participation in flagship international experiments
United Kingdom View profile
IOP Publishing
IOP Publishing is a wholly owned subsidiary of the Institute of Physics, a leading scientific society with a worldwide membership of physicists from all sectors
United Kingdom View profile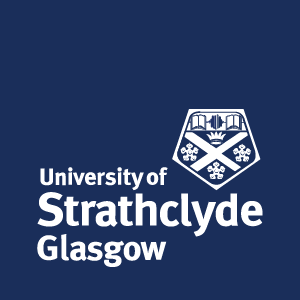
University of Strathclyde
Research is of central importance in everything we do. It informs our teaching and helps us to make a difference to business, industry and society as a whole. We're in the UK’s top 20 universities for research intensity according to the Times Higher Education’s analysis of REF2014. We are also delighted to have won Times Higher Education University of the Year 2019 – the only university to be awarded the UK-wide award for a second time
United Kingdom View profile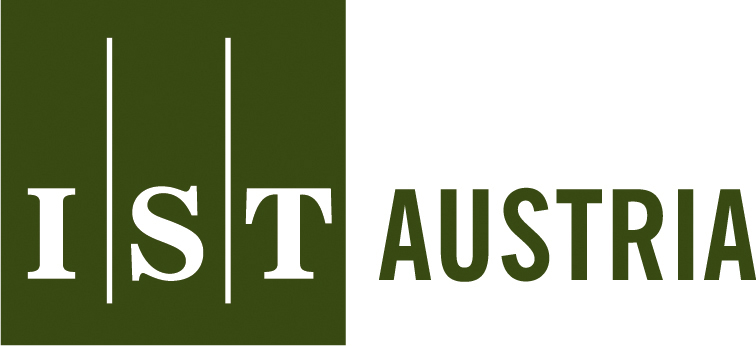
Institute of Science and Technology Austria
The Institute of Science and Technology Austria is an international research institute in natural and mathematical sciences, located in Maria Gugging, Klosterneuburg, 20 km north-west of the Austrian capital of Vienna
Austria View profile